Harnessing AI in Biotech: Transforming Drug Discovery for Arthritis Treatment
The Synergistic Power of Machine Learning and Data Science
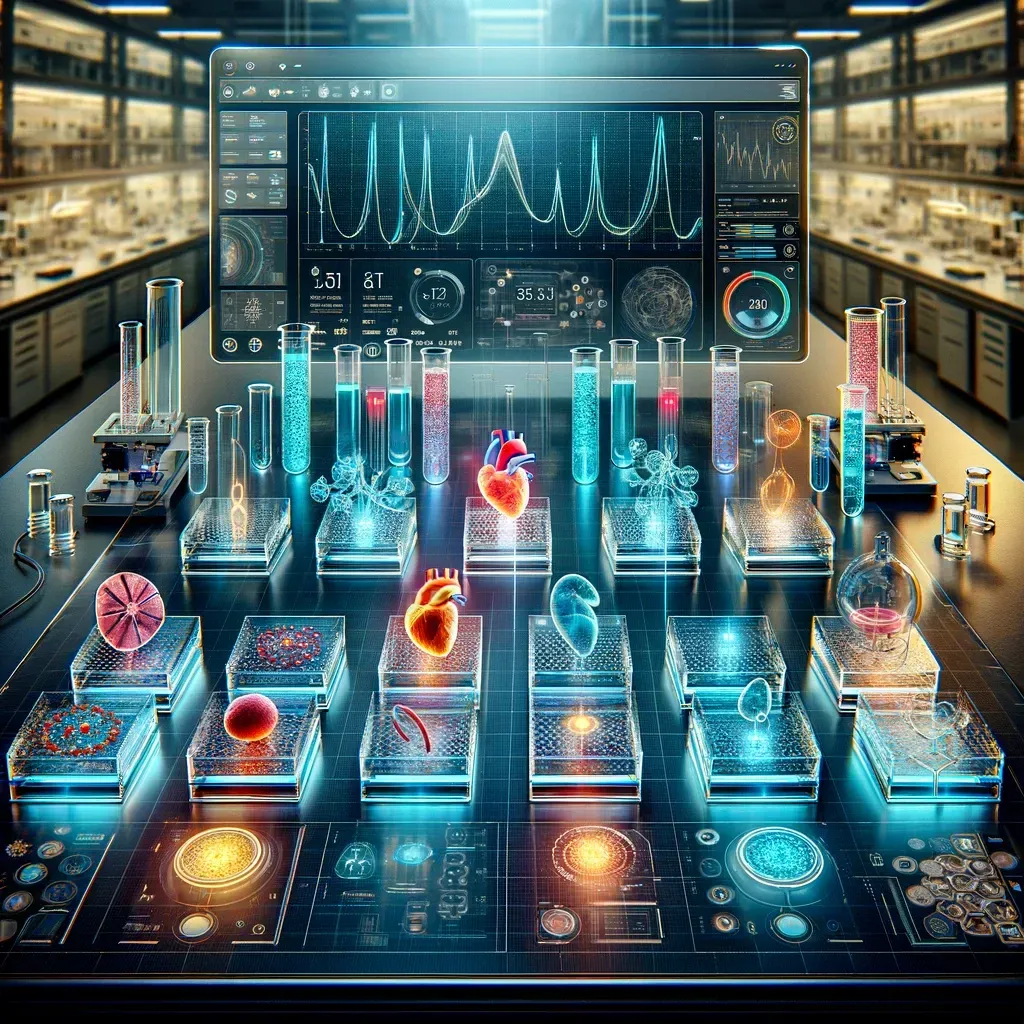
The quest for effective arthritis treatments faces the dual challenges of complexity and the need for precision. Artificial Intelligence (AI), specifically through the lenses of machine learning and data science, has emerged as a beacon of hope, offering innovative pathways to accelerate and refine drug discovery in biotech research.
Understanding AI in Biotech
AI encompasses a range of technologies, but for biotech research, machine learning and data science stand out. Machine learning, with its ability to learn from and make predictions on data, offers unprecedented opportunities to uncover new treatment pathways. Data science, the discipline of extracting knowledge and insights from structured and unstructured data, provides the necessary foundation for these predictions to be meaningful and actionable.
Machine Learning's Role in Arthritis Research
Machine learning techniques, such as deep learning, have been instrumental in identifying new drug targets and elucidating the complex mechanisms underlying arthritis. By analyzing patterns within large datasets, machine learning models can predict how different molecules might interact with biological pathways involved in arthritis, leading to potential new treatments. Success stories include the identification of novel biomarkers for disease progression and the discovery of compounds with therapeutic potential.
Data Science: The Backbone of AI-Driven Research
Data science plays a critical role in managing the vast amounts of biological and clinical data generated in arthritis research. Through sophisticated data management and analysis techniques, data scientists can uncover hidden insights within this data, providing a rich foundation for machine learning algorithms to build upon. These insights can lead to a deeper understanding of disease mechanisms and patient responses to treatment, ultimately guiding the development of more effective therapies.
Synergizing Machine Learning and Data Science
The interplay between machine learning and data science in biotech research is a game-changer. This synergy allows for the creation of predictive models that can simulate drug interactions within the human body more accurately, screen compounds for efficacy and safety more efficiently, and identify biomarkers indicative of treatment response. Such advancements are not only accelerating the drug discovery process but also making it more precise and personalized.
Challenges and Ethical Considerations
While the potential is immense, the application of AI in arthritis research is not without its challenges. Issues such as data quality, model interpretability, and the ethical implications of AI-driven decisions need careful consideration. Ensuring the privacy and security of patient data is paramount, as is maintaining transparency in how AI models make predictions and influence research directions.
Future Direction
As AI technologies, particularly machine learning and data science, continue to evolve, their impact on arthritis treatment research is expected to grow. Future advancements could lead to more sophisticated models capable of simulating the entire disease process or personalized medicine approaches that tailor treatments to individual genetic profiles. Collaborative efforts between biotechnologists, data scientists, and machine learning experts will be crucial in realizing these possibilities. The journey from data to discovery is being reshaped, promising a future where arthritis patients can look forward to more effective and personalized treatment options.
Biotech News
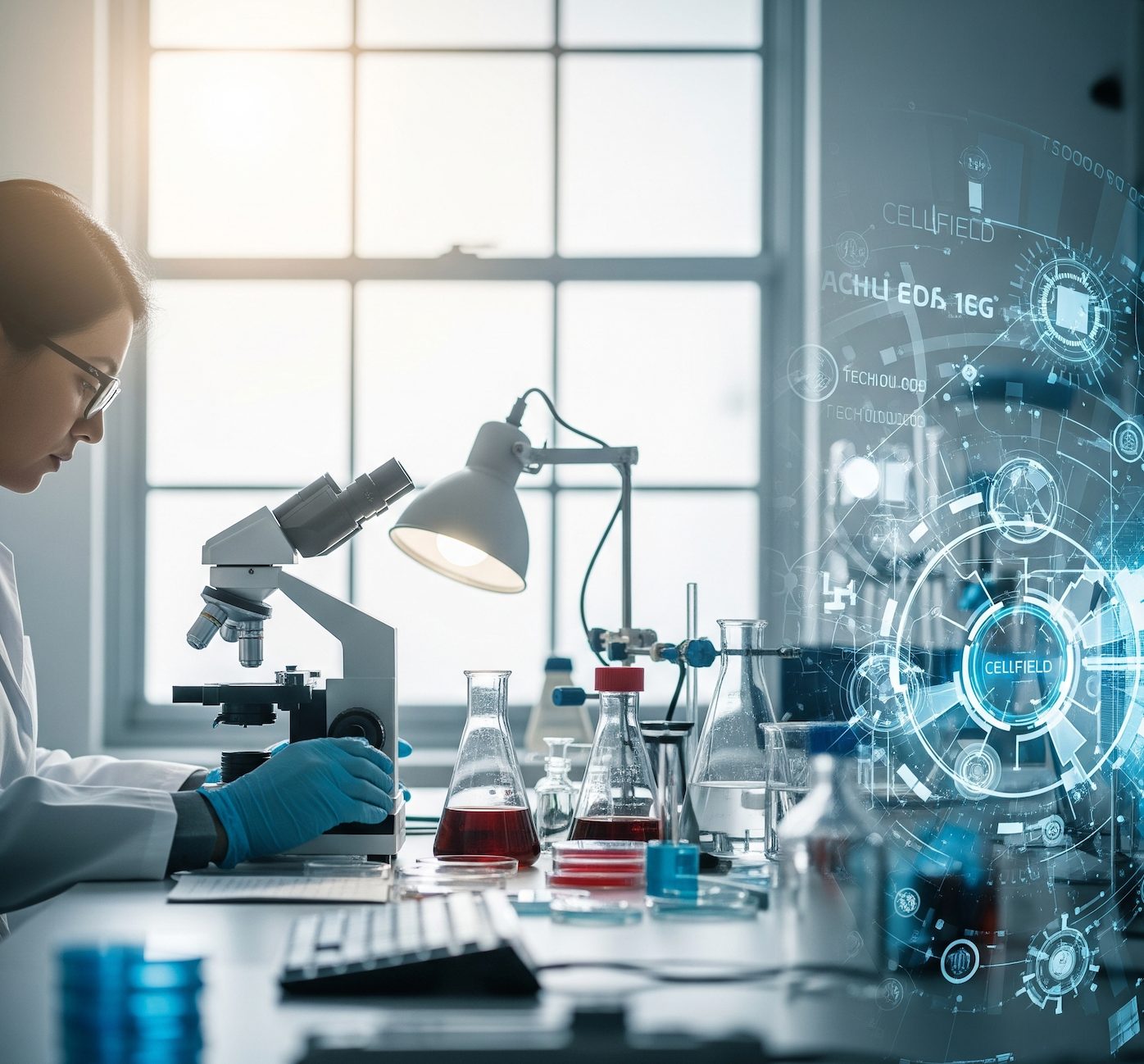
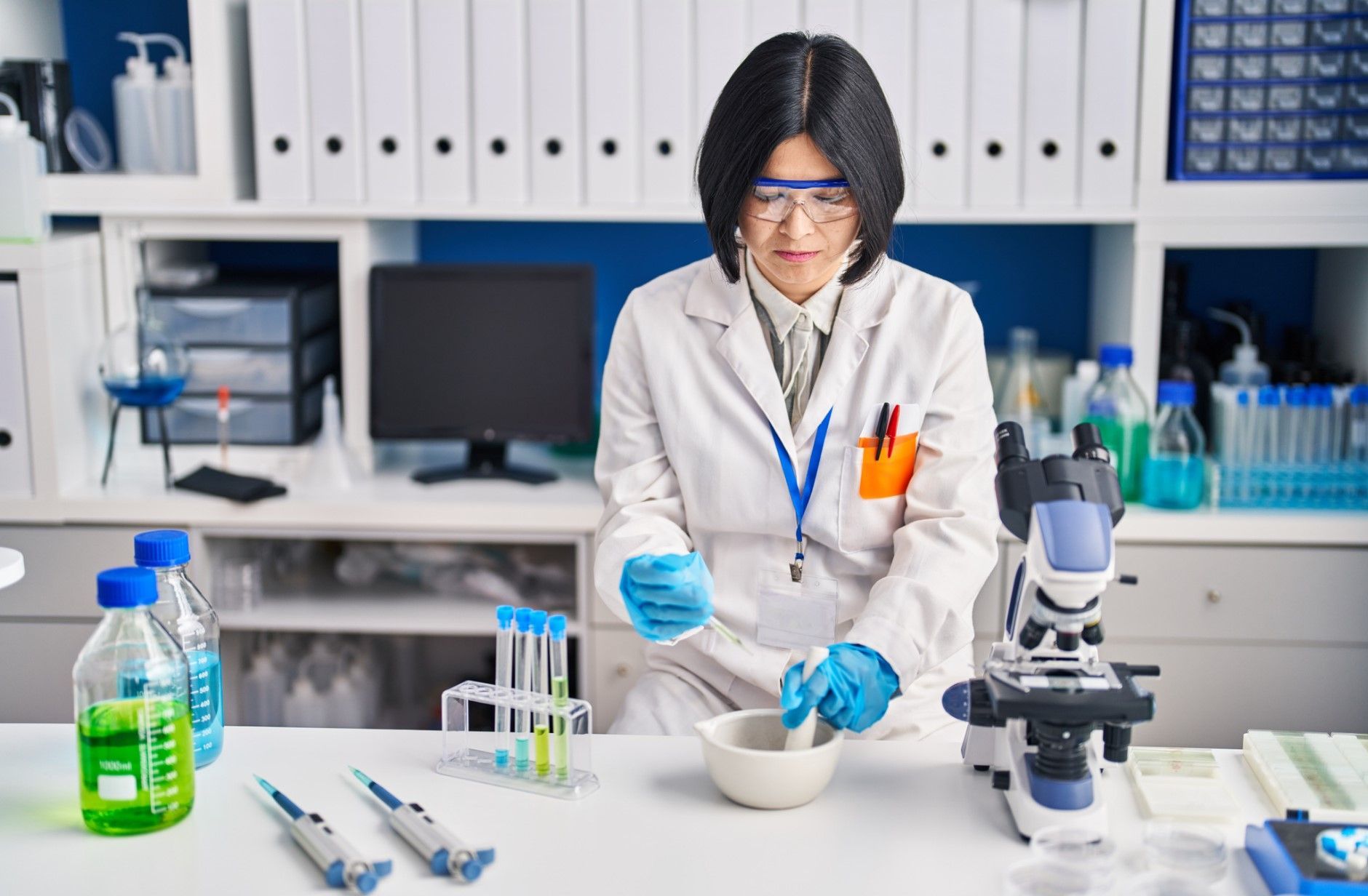